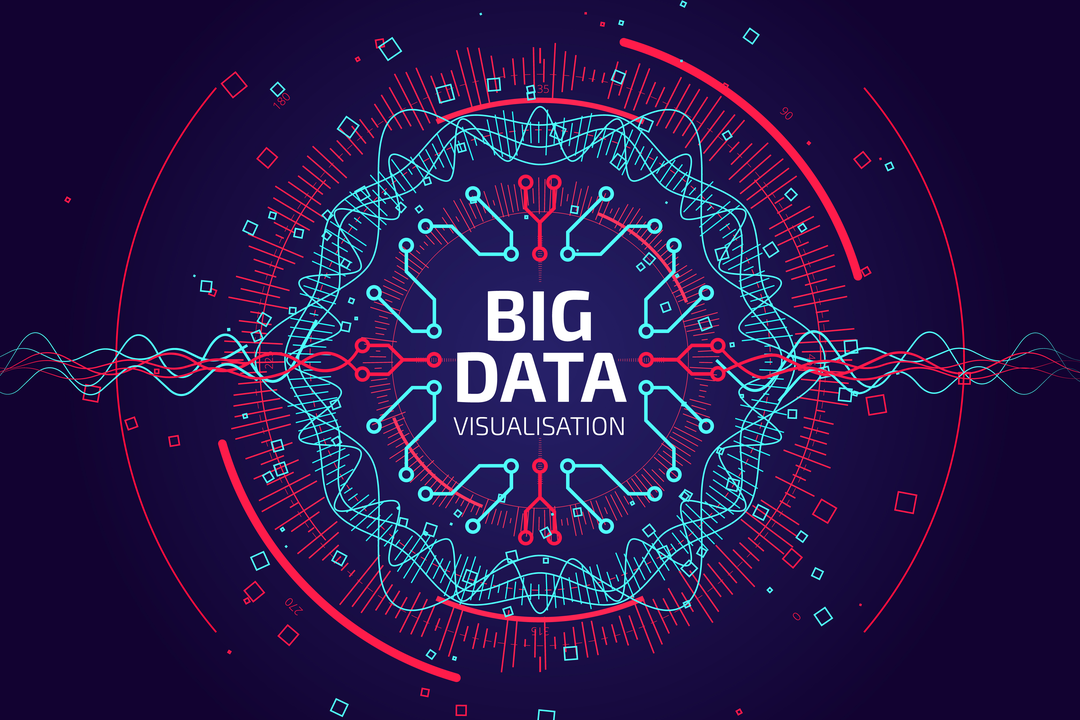
In as much RPA has gained significant acceptance across sectors; Big Data is another digital concept that has garnered major mindshare amongst large enterprises. The reason for Big Data’s success as a technological concept is its ability to handle 3Vs of data very effectively – Volume, Velocity, and Variety.
Every organization and its eco-system generate huge amounts of transaction data (predominantly structured data) and interaction data (predominantly semi and unstructured data such as emails, social posts, voice, video, images, etc), at an alarming rate, which upon analysis, could throw up wonderful hindsight (Descriptive Analytics), Insights (Diagnostic Analytics) and Foresights (Predictive Analytics).
While the business case for Big Data is compelling, the technology associated with compiling all types of data – structured, semi-structured, and structured into a common database is complex. Only after extracting, cleansing, validating, and loading all types of data into a database, which is referred to as Data Engineering activity, one can apply appropriate algorithms to arrive at the right insights or foresight (Referred to as Data science).
Despite the wonderful ETL tools that are available in the market, there is a significant amount of manual activity that happens around data cleansing, validation, and compilation of what is called data federation. This is where RPA plus AI can be of big help.
BOTs do not essentially improve analytics capabilities but aid in data collection. The core benefit of RPA in regards to analytics is in data federation: the capability to collect data from many different sources and aggregate it in an easy-to-analyze format.
Imagine a big data scenario wherein an auto insurer can generate estimates that include repair methods, times, and spare parts costs by just uploading images of the damaged vehicle. This means the algorithm is able to learn from the history of similar such damages and associated repair estimates. To develop a solution such as this, one must ensure the availability of structured data from ERP and Maintenance systems and unstructured data such as images in the right formats. Compiling this information from various systems, cleaning and validating them before loading them to the database involves a lot of manual work despite having the right ETL tools. RPA can do this task at fraction of time and cost.
In summary, if you are considering a Big Data solution, please have RPA as one of its critical solution components. This would save nearly 20-30% of manual efforts associated with Data Engineering.
RPA and Big Data are indeed a perfect match.